TrustMAE: A Noise-Resilient Defect Classification Framework using Memory-Augmented Auto-Encoders with Trust Regions
IEEE Workshop on Applications of Computer Vision (WACV)
Authors
Daniel Stanley Tan, Yi-Chun Chen, Trista Pei-Chun Chen, Wei-Chao Chen
Published
2021/6/14
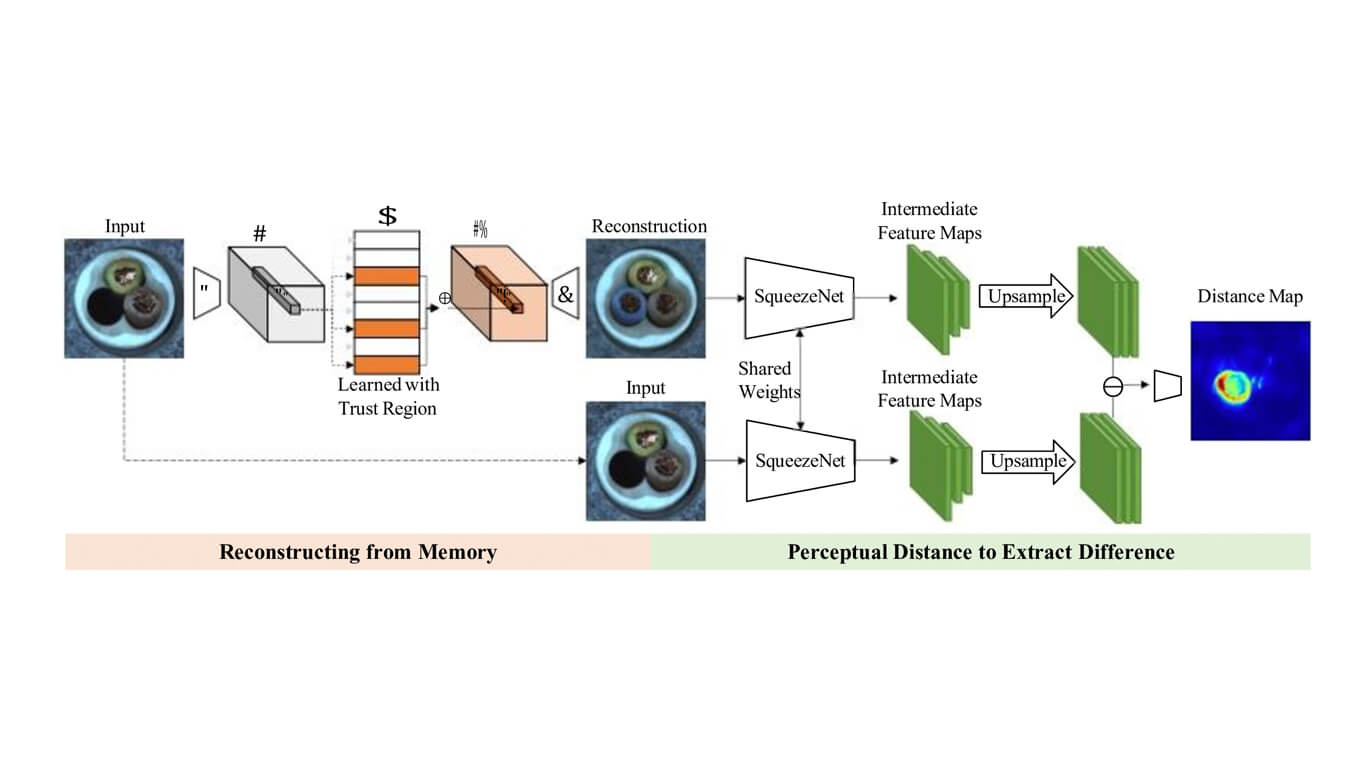
Abstract
In this paper, we propose a framework called Trust MAE to address the problem of product defect classification. Instead of relying on defective images that are difficult to collect and laborious to label, our framework can accept datasets with unlabeled images. Moreover, unlike most anomaly detection methods, our approach is robust against noises, or defective images, in the training dataset.
Our framework uses a memory-augmented auto-encoder with a sparse memory addressing scheme to avoid over generalizing the auto-encoder, and a novel trust-region memory updating scheme to keep the noises away from the memory slots. The result is a framework that can reconstruct defect-free images and identify the defective regions using a perceptual distance network. When compared against various state-of-the-art baselines, our approach performs competitively under noise-free MVTec datasets. More importantly, it remains effective at a noise level up to 40% while significantly outperforming other baselines.
Keywords
- Computer Vision and Pattern Recognition
- Anomaly Detection
- AutoEncoder