A Mixed-Domain Self-Attention Network for Multilabel Cardiac Irregularity Classification Using Reduced-Lead Electrocardiogram
IEEE Computers in Cardiology 2021
Authors
Hao-Chun Yang, Wan-Ting Hsieh, Trista Pei-Chun Chen
Published
2022/1/10
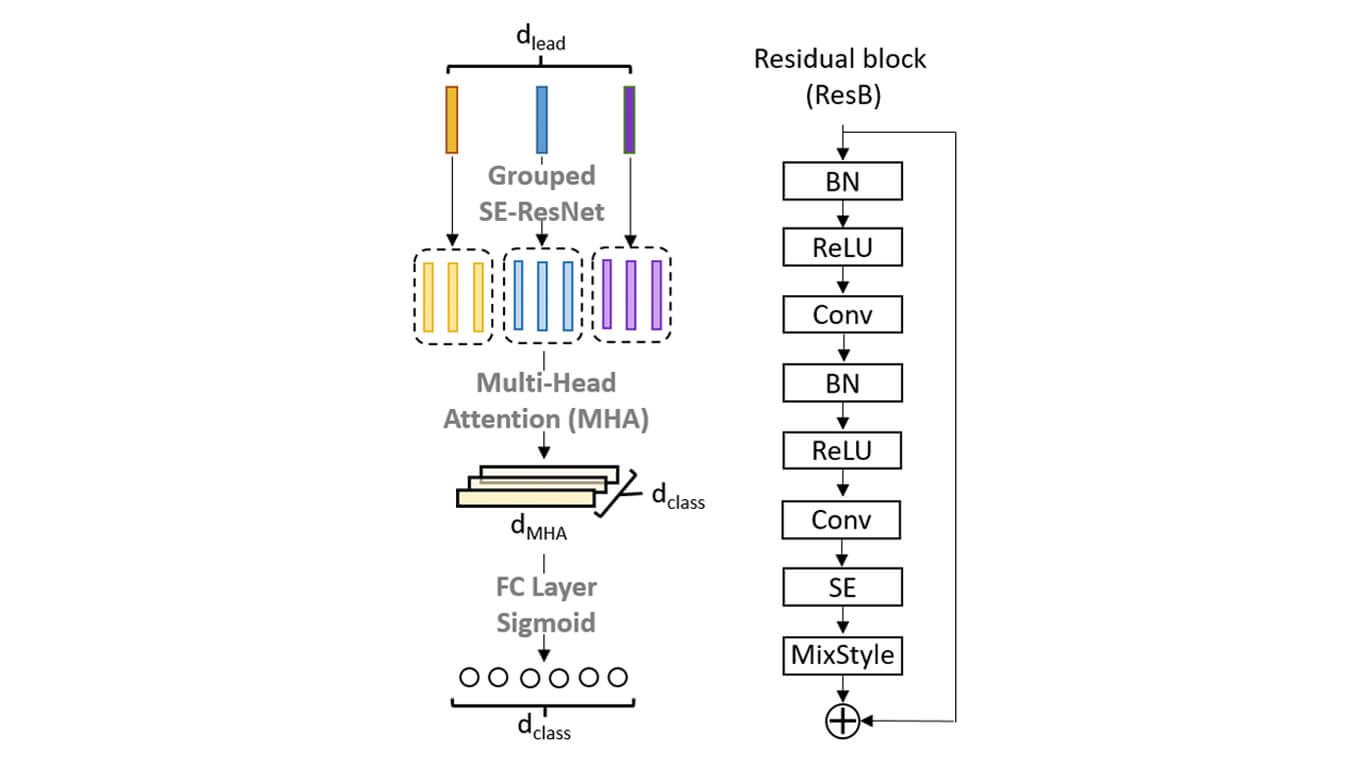
Abstract
Electrocardiogram(ECG) is commonly used to detect cardiac irregularities such as atrial fibrillation, bradycardia, and other irregular complexes. While previous studies have achieved great accomplishment classifying these irregularities with standard 12-lead ECGs, there existed limited evidence demonstrating the utility of reduced-lead ECGs in capturing a wide-range of diagnostic information. In addition, classification model’s generalizability across multiple recording sources also remained uncovered.
As part of the PhysioNet Computing in Cardiology Challenge 2021, our team HaoWan AIeC, proposed Mixed-Domain Self-Attention Resnet (MDARsn) to identify cardiac abnormalities from reduced-lead ECG. Our classifiers received scores of 0.602, 0.593, 0.597, 0.591, and 0.589 (ranked 54th, 37th, 38th, 38th, and 39th) for the 12-lead, 6-lead, 4-lead, 3-lead, and 2-lead versions of the hidden validation set with the evaluation metric defined by the challenge.
Keywords
- Domain Generalization
- Self-Attention