Development of a Deep Learning-Based Tool to Assist Wound Classification
Journal of Plastic, Reconstructive & Aesthetic Surgery
Authors
Po-Hsuan Huang, Yi-Hsiang Pan, Ying-Sheng Luo, Yi-Fan Chen, Yu-Cheng Lo, Trista Pei-Chun Chen, Cherng-Kang Perng
Published
2023/2/8
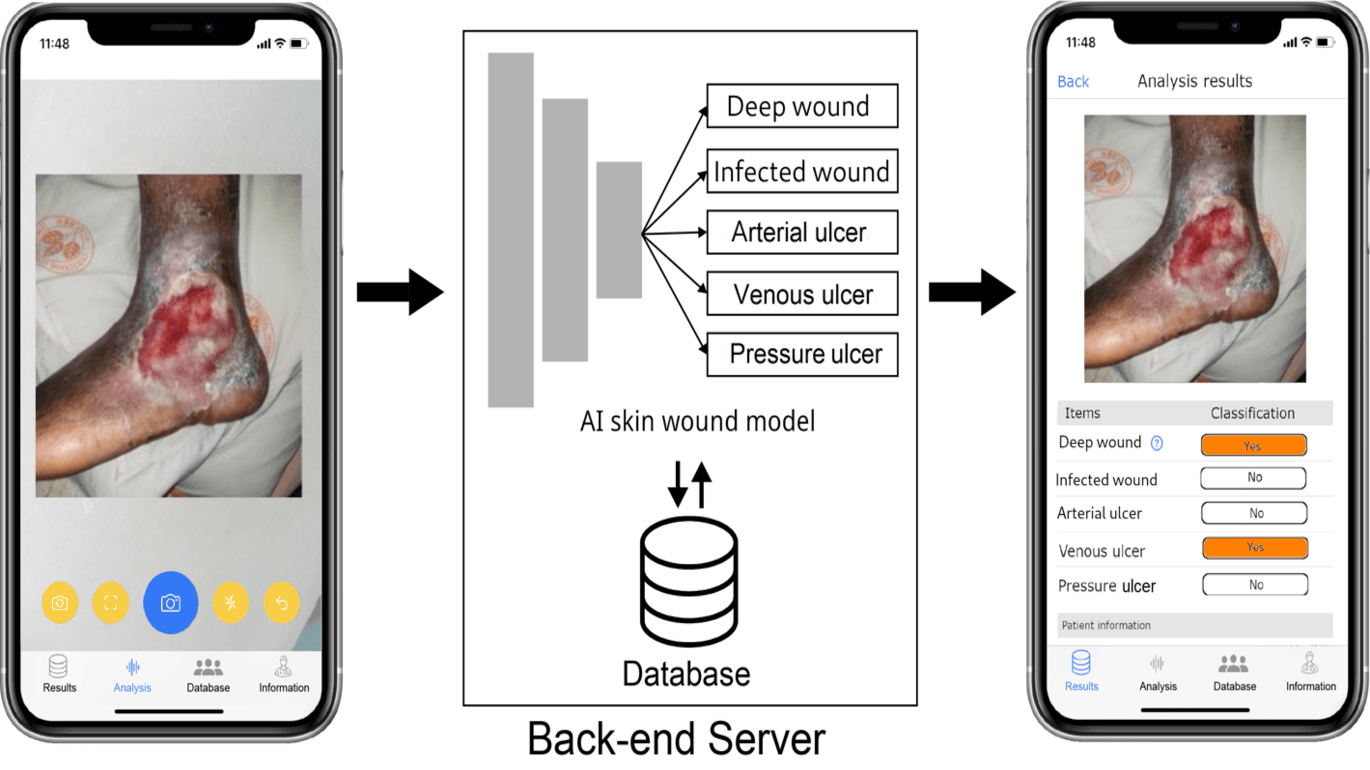
Abstract
This paper presents a deep learning-based wound classification tool that can assist medical personnel in non-wound care specialization to classify five key wound conditions, namely deep wound, infected wound, arterial wound, venous wound, and pressure wound, given color images captured using readily available cameras. The accuracy of the classification is vital for appropriate wound management. The proposed wound classification method adopts a multi-task deep learning framework that leverages the relationships among the five key wound conditions for a unified wound classification architecture. With differences in Cohen’s kappa coefficients as the metrics to compare our proposed model with humans, the performance of our model was better or non-inferior to those of all human medical personnel. Our convolutional neural network-based model is the first to classify five tasks of deep, infected, arterial, venous, and pressure wounds simultaneously with good accuracy. The proposed model is compact and matches or exceeds the performance of human doctors and nurses. Medical personnel who do not specialize in wound care can potentially benefit from an app equipped with the proposed deep learning model.
Keywords
- Deep Learning
- Wounds and Injuries
- Wound infection