Benchmarking Smoothness and Reducing High-Frequency Oscillations in Continuous Control Policies
IEEE/RSJ International Conference on Intelligent Robots and Systems (IROS 2024)
Authors
Guilherme Christmann*, Ying-Sheng Luo*, Hanjaya Mandala*, and Wei-Chao Chen
Published
2024/10/22
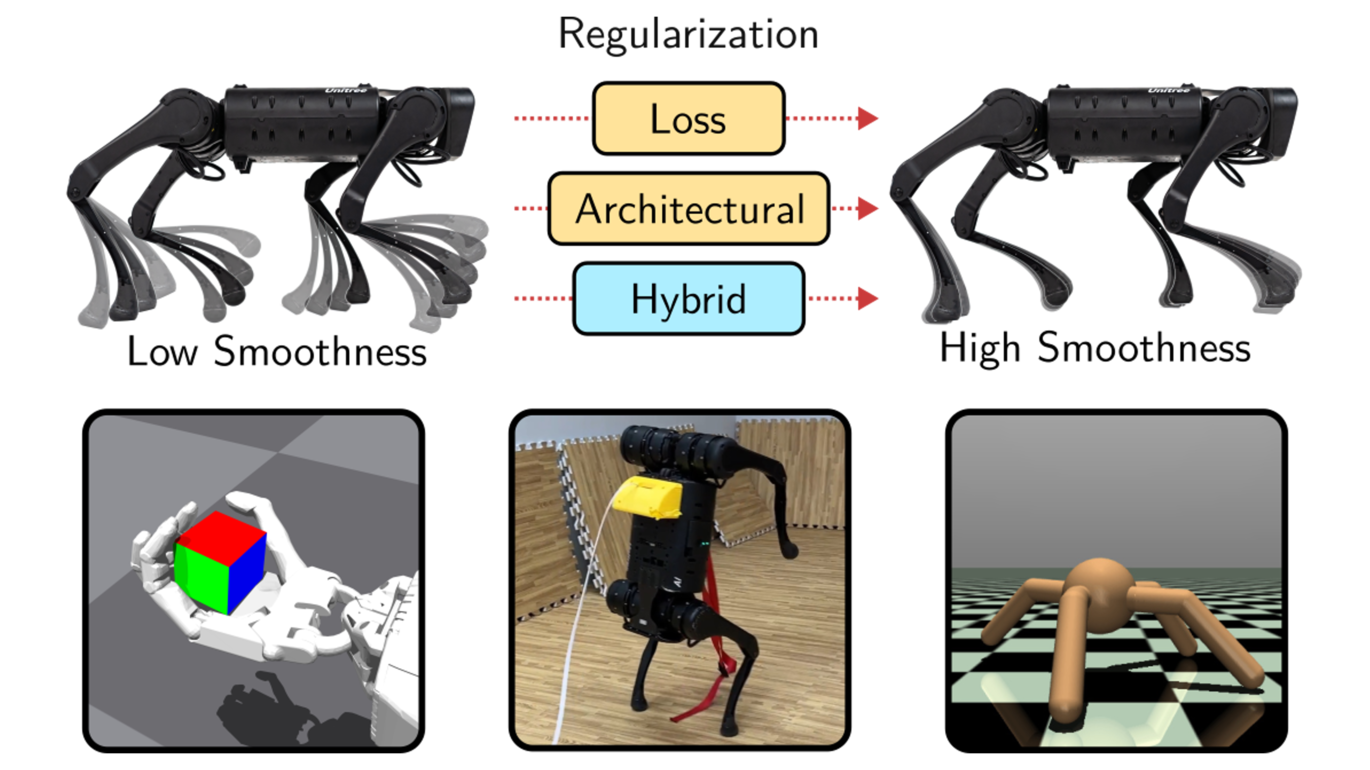
Abstract
Reinforcement learning (RL) policies are prone to high-frequency oscillations, especially undesirable when deploying to hardware in the real-world. In this paper, we identify, categorize, and compare methods from the literature that aim to mitigate high-frequency oscillations in deep RL. We define two broad classes: loss regularization and architectural methods. At their core, these methods incentivize learning a smooth mapping, such that nearby states in the input space produce nearby actions in the output space.
We present benchmarks in terms of policy performance and control smoothness on traditional RL environments from the Gymnasium and a complex manipulation task, as well as three robotics locomotion tasks that include deployment and evaluation with real-world hardware. Finally, we also propose hybrid methods that combine elements from both loss regularization and architectural methods. We find that the best-performing hybrid outperforms other methods, and improves control smoothness by 26.8% over the baseline, with a worst-case performance degradation of just 2.8%.
Keywords
- Robotics
- Reinforcement Learning