A Robust Collaborative Learning Framework Using Data Digests and Synonyms to Represent Absent Clients
IEEE International Conference on Multimedia Information Processing and Retrieval (IEEE MIPR 2021)
Authors
Chih-Fan Hsu, Ming-Ching Chang, and Wei-Chao Chen
Published
2022/9/8
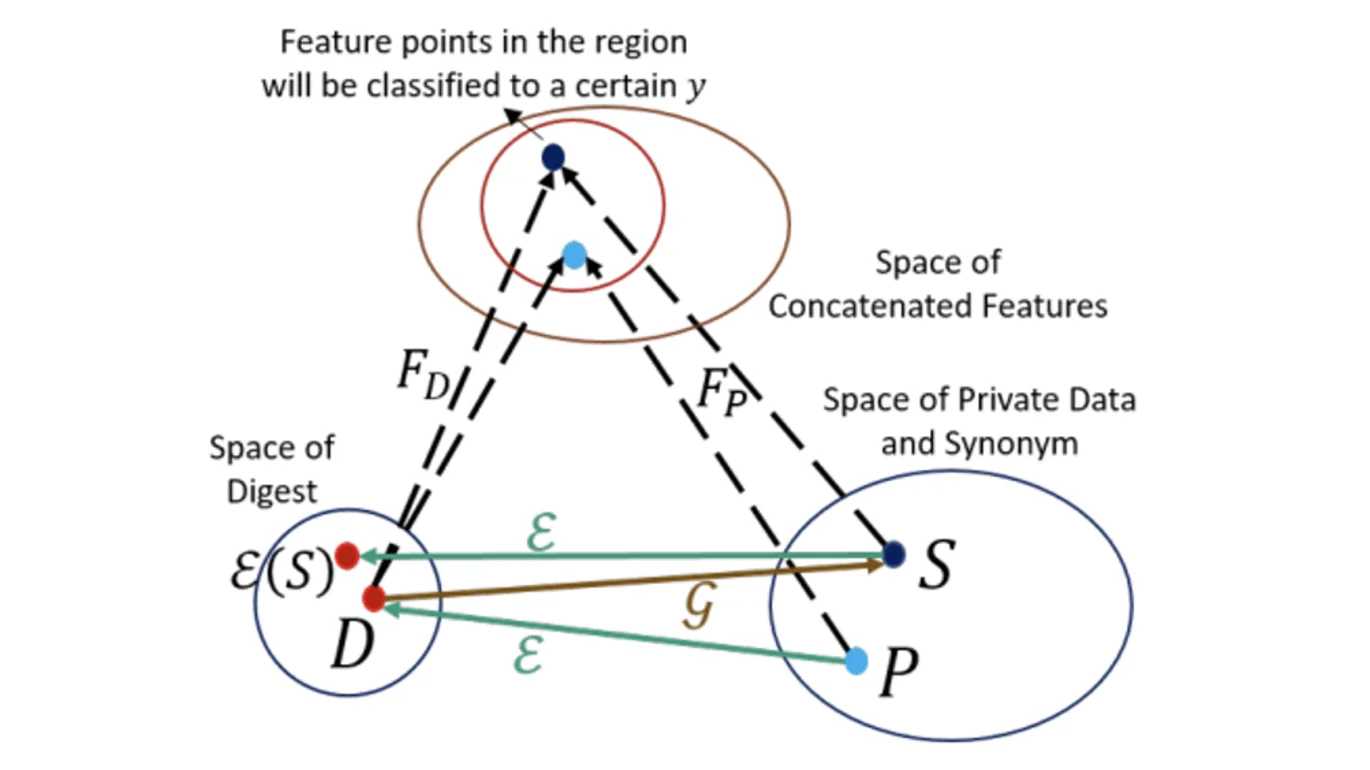
Abstract
We propose Collaborative Learning with Synonyms (CLSyn), a robust and versatile collaborative machine learning framework that can tolerate unexpected client absence during training while maintaining high model accuracy.
Client absence during collaborative training can seriously degrade model performances, particularly for unbalanced and non-IID client data.
We address this issue by introducing the notion of data digests of the training samples from the clients.
The expansion of digests called synonyms can represent the original samples on the server and thus maintain overall model accuracy, even after the clients become unavailable.
We compare our CLSyn implementations against three centralized Federated Learning algorithms, namely FedAvg, FedProx, and FedNova as baselines.
Results on CIFAR-10, CIFAR-100, and EMNIST show that CLSyn consistently outperforms these baselines by significant margins in various client absence scenarios.
Keywords
- Federated Learning
- Data digest
- Privacy