A Trainable Reconciliation Method for Hierarchical Time-Series
ITISE (7th International Conference on Time Series and Forecasting)
Authors
Davide Burba, Trista Chen
Published
2021/1/5
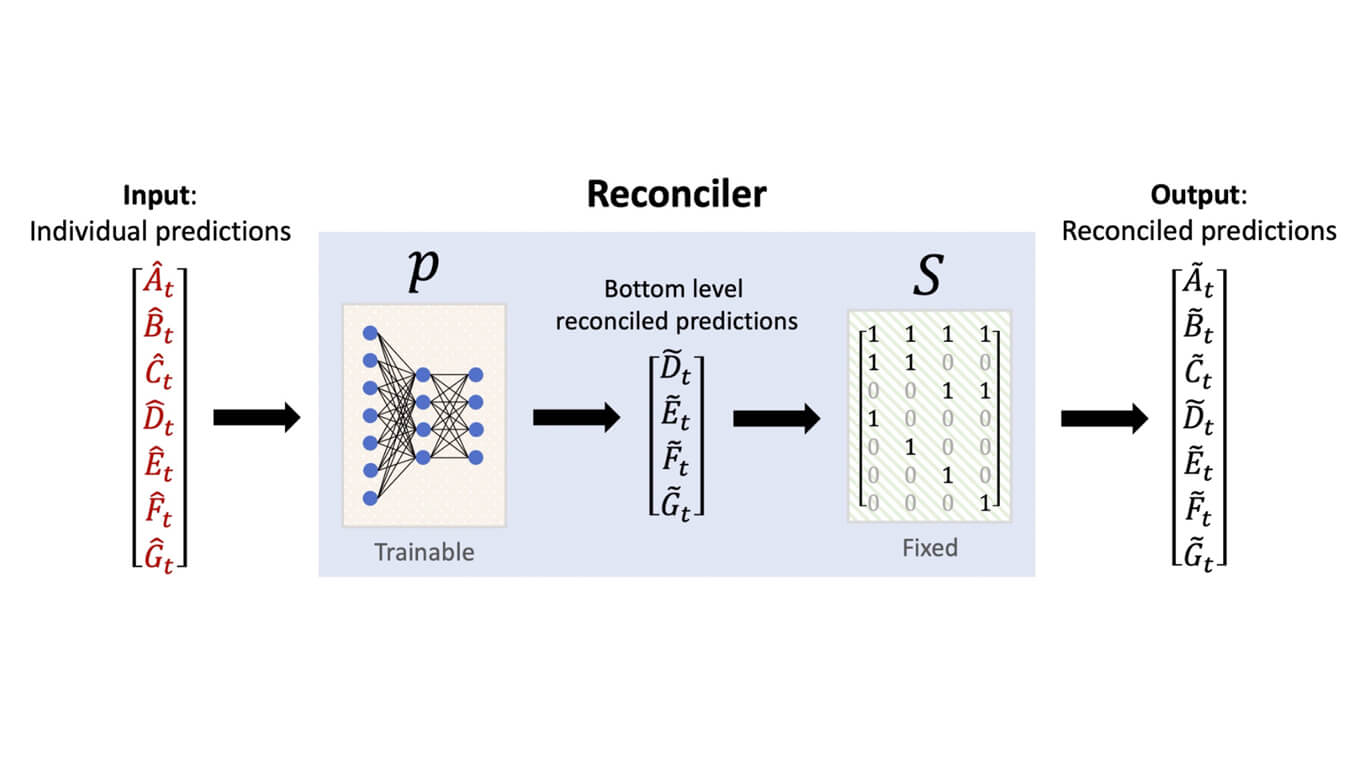
Abstract
In numerous applications, it is required to produce forecasts for multiple time-series at different hierarchy levels. An obvious example is given by the supply chain in which demand forecasting may be needed at a store, city, or country level. The independent forecasts typically do not add up properly because of the hierarchical constraints, so a reconciliation step is needed.
In this paper, we propose a new general, flexible, and easy-to-implement reconciliation strategy based on an encoder-decoder neural network. By testing our method on four real world datasets, we show that it can consistently reach or surpass the performance of existing methods in the reconciliation setting.
Keywords
- Hierarchical Time-Series
- Neural Networks
- Reconciliation
- Forecasting