Profiling Patient Transcript Using Large Language Model Reasoning Augmentation for Alzheimer’s Disease Detection
46th Annual International Conference of the IEEE Engineering in Medicine and Biology Society (EMBC 2024)
作者
Chin-Po Chen, Jeng-Lin Li
發表日期
2024/4/29
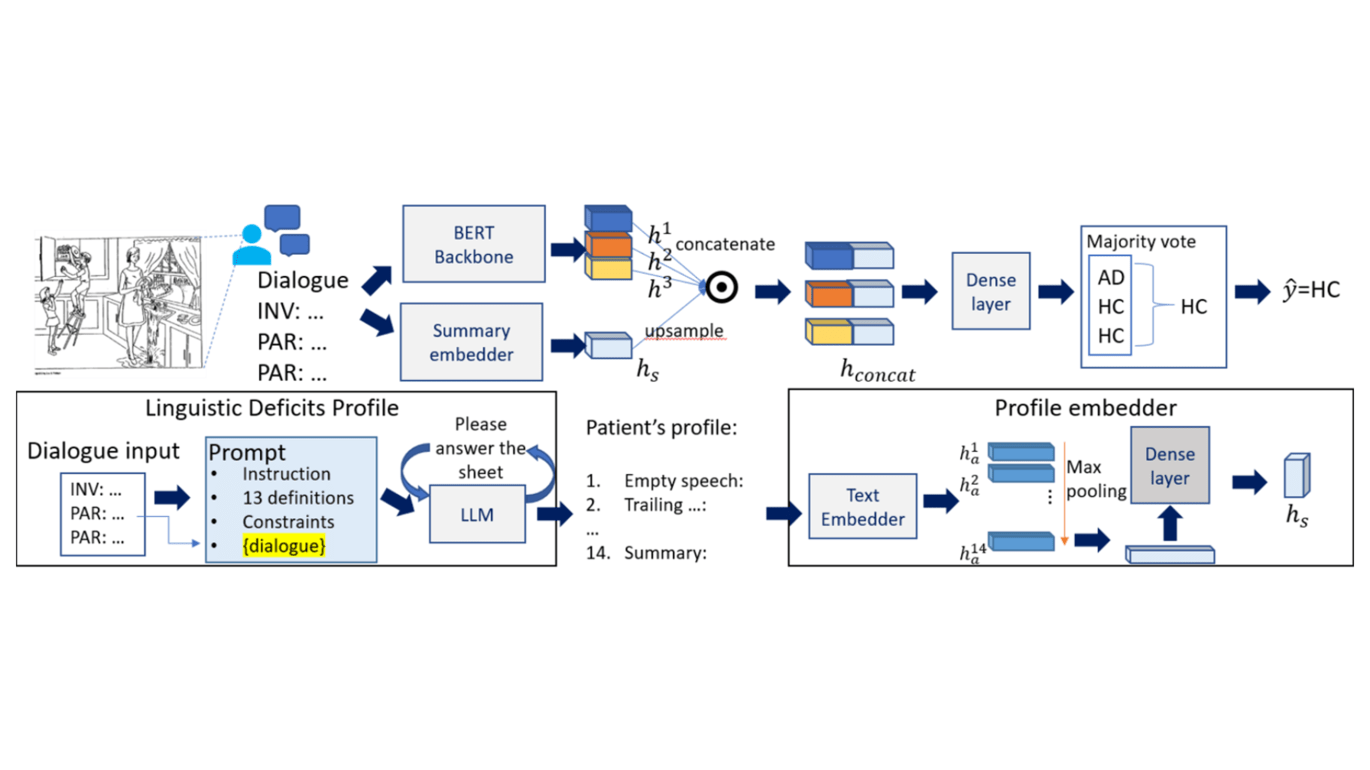
概要
Alzheimer’s disease (AD) stands as the predominant cause of dementia, characterized by a gradual decline in speech and language capabilities. Recent deeplearning advancements have facilitated automated AD detection through spontaneous speech. However, common transcript-based detection methods directly model text patterns in each utterance without a global view of the patient’s linguistic characteristics, resulting in limited discriminability and interpretability. Despite the enhanced reasoning abilities of large language models (LLMs), there remains a gap in fully harnessing the reasoning ability to facilitate AD detection and model interpretation. Therefore, we propose a patient-level transcript profiling framework leveraging LLM-based reasoning augmentation to systematically elicit linguistic deficit attributes.
The summarized embeddings of the attributes are integrated into an Albert model for AD detection. The framework achieves 8.51% ACC and 8.34% F1 improvements on the ADReSS dataset compared to the baseline without reasoning augmentation. Our further analysis shows the effectiveness of our identified linguistic deficit attributes and the potential to use LLM for AD detection interpretation.
關鍵字
- Large langauge model
- Alzheimer’s Disease
- Language assessemt