Learning Multi-Manifold Embedding for Out-Of-Distribution Detection
2024 European Conference on Computer Vision Workshop (ECCVW2024)
作者
Jeng-Lin Li, Ming-Ching Chang, and Wei-Chao Chen
發表日期
2024/9/29
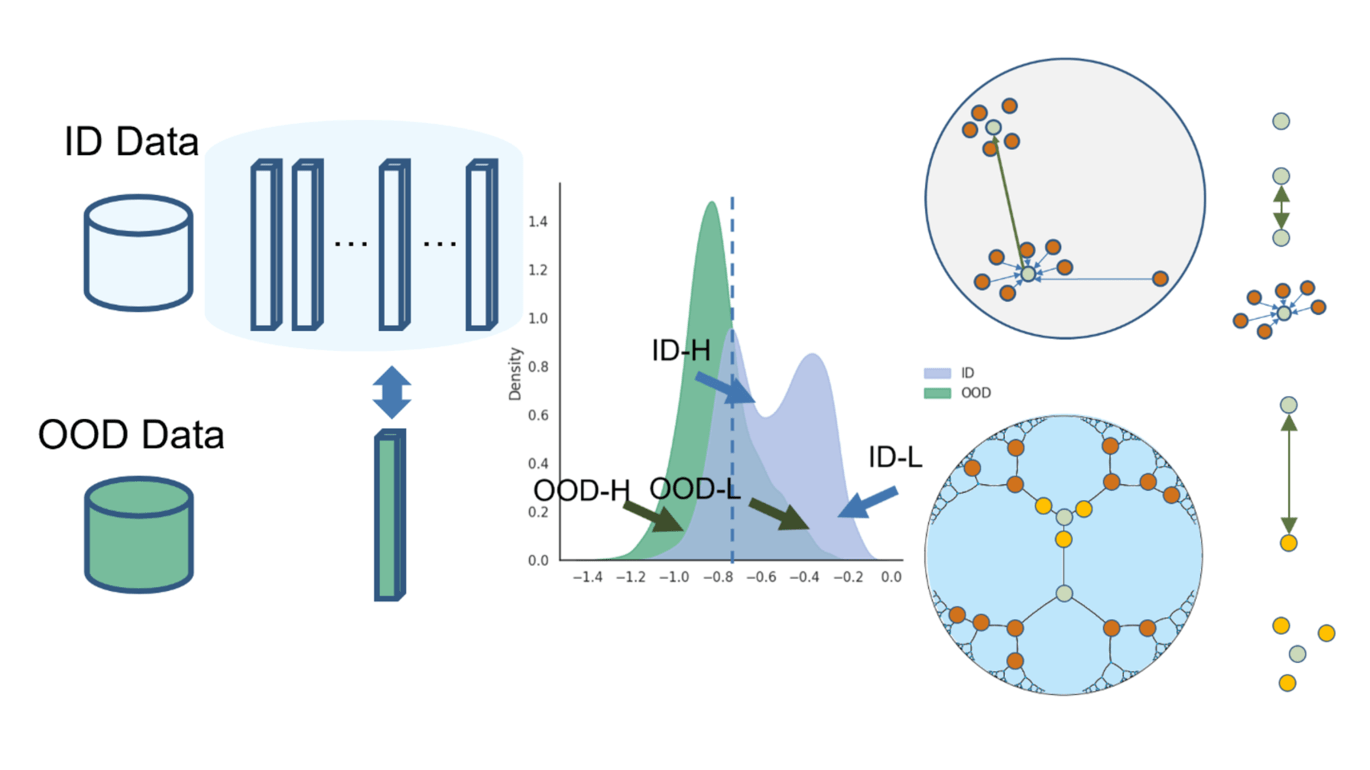
概要
Detecting out-of-distribution (OOD) samples is crucial for trustworthy AI in real-world applications. Leveraging recent advances in representation learning and latent embeddings, Various scoring algorithms estimate distributions beyond the training data. However, a single embedding space falls short in characterizing in-distribution data and defending against diverse OOD conditions.
This paper introduces a novel Multi-Manifold Embedding Learning (MMEL) framework, optimizing hypersphere and hyperbolic spaces jointly for enhanced OOD detection. MMEL generates representative embeddings and employs a prototype-aware scoring function to differentiate OOD samples.
Experiments on six open datasets demonstrate MMEL’s significant reduction in FPR while maintaining a high AUC compared to state-of-the-art distance-based OOD detection methods.
Notably, enrolling ten OOD samples without retraining achieves comparable FPR and AUC to modern outlier exposure methods using 80 million outlier samples for model training.
關鍵字
- Out-of-distribution detection
- Hyperbolic
- Multiple manifold learning
- Hypersphere