FedTrust: Towards Building
Secure Robust and Trustworthy Moderators for Federated Learning
IEEE International Conference on Multimedia Information Processing and Retrieval (IEEE MIPR 2021)
作者
Chih-Fan Hsu, Jing-Lun Huang, Feng-Hao Liu, Ming-Ching Chang, and Wei-Chao Chen
發表日期
2022/9/8
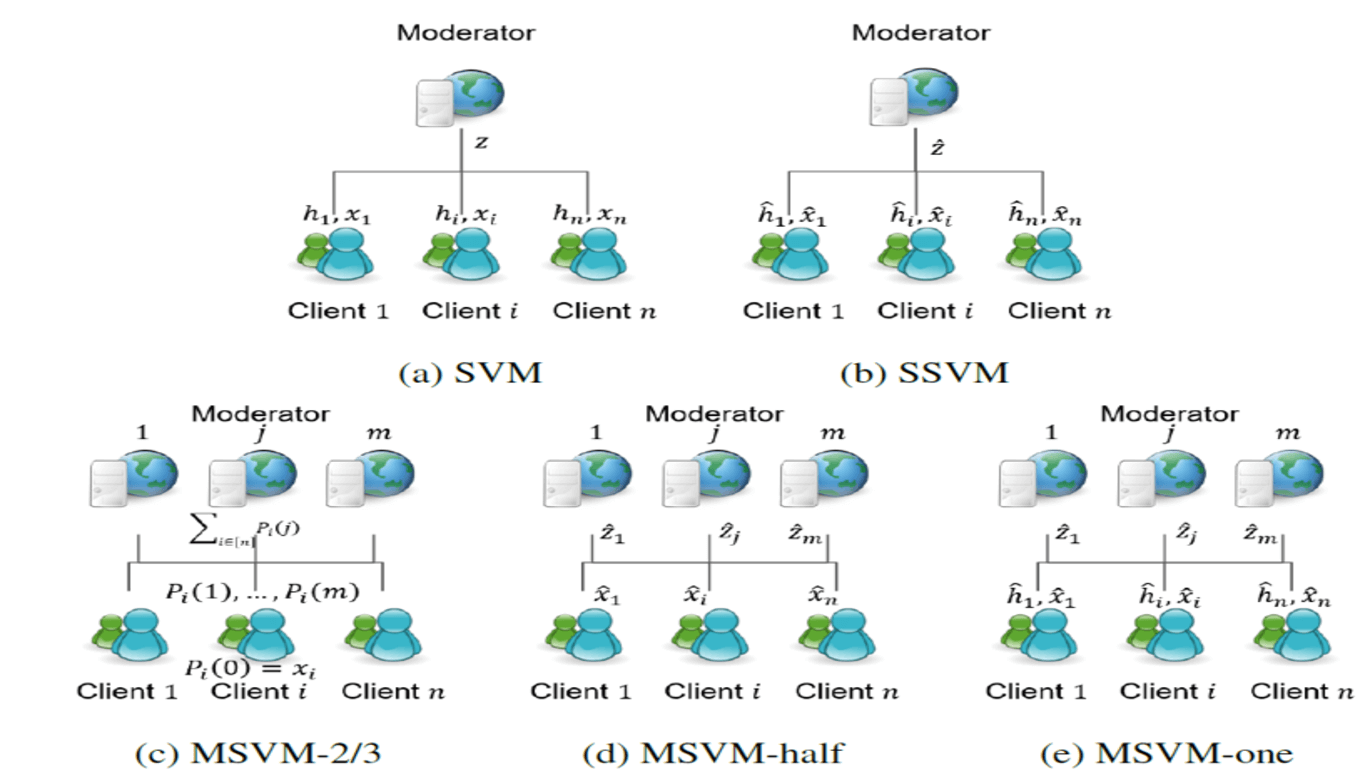
概要
Most Federated Learning (FL) systems are built upon a strong assumption of trust—clients fully trust the centralized moderator, which might not be feasible in practice.
This work aims to mitigate the assumption by using appropriate cryptographic tools.
Particularly, we examine various scenarios with different trust demands in FL, and then design the corresponding practical protocols with lightweight cryptographic tools.
Three solutions for secure and trustworthy aggregation are proposed with increasing sophistication: (1) a single verifiable moderator, (2) a single secure and verifiable moderator, and (3) multiple secure and verifiable moderators, which can handle adversarial behaviors with different levels.
We evaluate the performances of all our proposed protocols on the test accuracy and the training time, showing that our protocols maintain the accuracy with time overhead from 30% to 156% depending on the secure and trustworthy levels. The protocols can be deployed in many practical FL settings with appropriate optimizations.
關鍵字
- Federated Learning
- Multi-Party Computation
- Homomorphic Encryption