Interpretable Estimation of the risk of Heart Failure Hospitalization from a 30-second Electrocardiogram
IEEE International Conference on E-Health and Bioengineering Conference (EHB 2022)
作者
Sergio Gonzalez, Wan-Ting Hsieh, Davide Burba, Trista Pei-Chun Chen, Chun-Li Wang, Victor Chien-Chia, Shang-Hung Chang
發表日期
2023/1/2
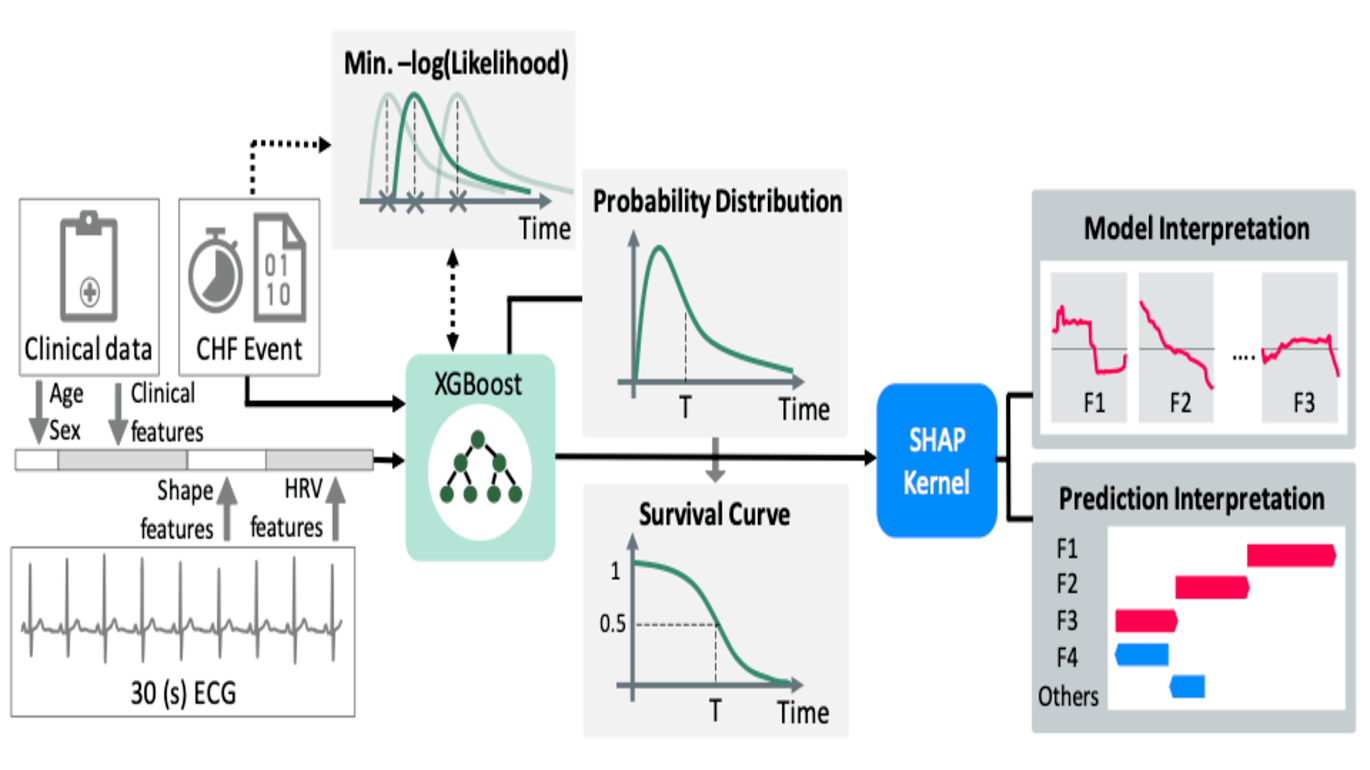
概要
Survival modeling in healthcare relies on explainable statistical models; yet, their underlying assumptions are often simplistic and, thus, unrealistic. Machine learning models can estimate more complex relationships and lead to more accurate predictions, but are non-interpretable. This study shows it is possible to estimate hospitalization for congestive heart failure by a 30 seconds single-lead electrocardiogram signal. Using a machine learning approach not only results in greater predictive power but also provides clinically meaningful interpretations. We train an XGBoost accelerated failure time model and exploit SHapley Additive exPlanations values to explain the effect of each feature on predictions. Our model achieved a concordance index of 0.828 and an area under the curve of 0.853 at one year and 0.858 at two years on a held-out test set of 6,573 patients. These results show that a rapid test based on an electrocardiogram could be crucial in targeting and treating high-risk individuals.
關鍵字
- Survival Qnalysis
- Heart Failure
- Interpretable AI
- XGBoost
- ECG