Improving Limited Supervised Foot Ulcer Segmentation Using Cross-Domain Augmentation
2024 IEEE International Conference on Acoustics, Speech, and Signal Processing (ICASSP 2024)
作者
Shang-Jui Kuo, Po-Han Huang, Chia-Ching Lin, Jeng-Lin Li, and Ming-Ching Chang
發表日期
2024/3/15
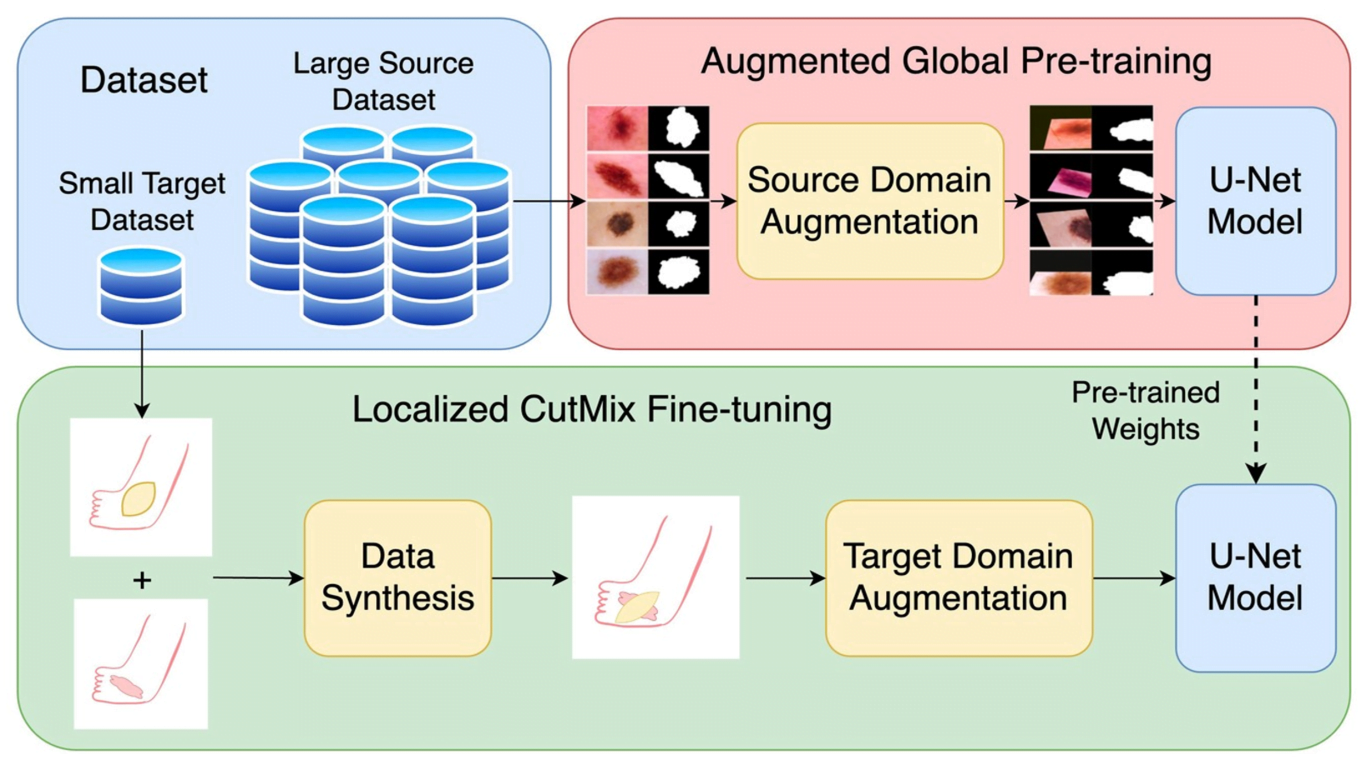
概要
Diabetic foot ulcers pose health risks, including higher morbidity, mortality, and amputation rates. Monitoring wound areas is crucial for proper care, but manual segmentation is subjective due to complex wound features and background variation. Expert annotations are costly and time-intensive, thus hampering large dataset creation. Existing segmentation models relying on extensive annotations are impractical in real-world scenarios with limited annotated data.
In this paper, we propose a cross-domain augmentation method named TransMix that combines Augmented Global Pre-training AGP and Localized CutMix Fine-tuning LCF to enrich wound segmentation data for model learning. TransMix can effectively improve the foot ulcer segmentation model training by leveraging other dermatology datasets not on ulcer skins or wounds. AGP effectively increases the overall image variability, while LCF increases the diversity of wound regions.
Experimental results show that TransMix increases the variability of wound regions and substantially improves the Dice score for models trained with only 40 annotated images under various proportions.
關鍵字
- Foot ulcer segmentation
- Transfer learning
- Data augmentation
- Pre-training
- CutMix