Expanding Versatility of Agile Locomotion through Policy Transitions Using Latent State Representation
International Conference on Robotics and Automation 2023 (ICRA 2023)
作者
Guilherme Christmann*, Ying-Sheng Luo*, Jonathan Hans Soeseno*, Wei-Chao Chen
发表日期
May-29
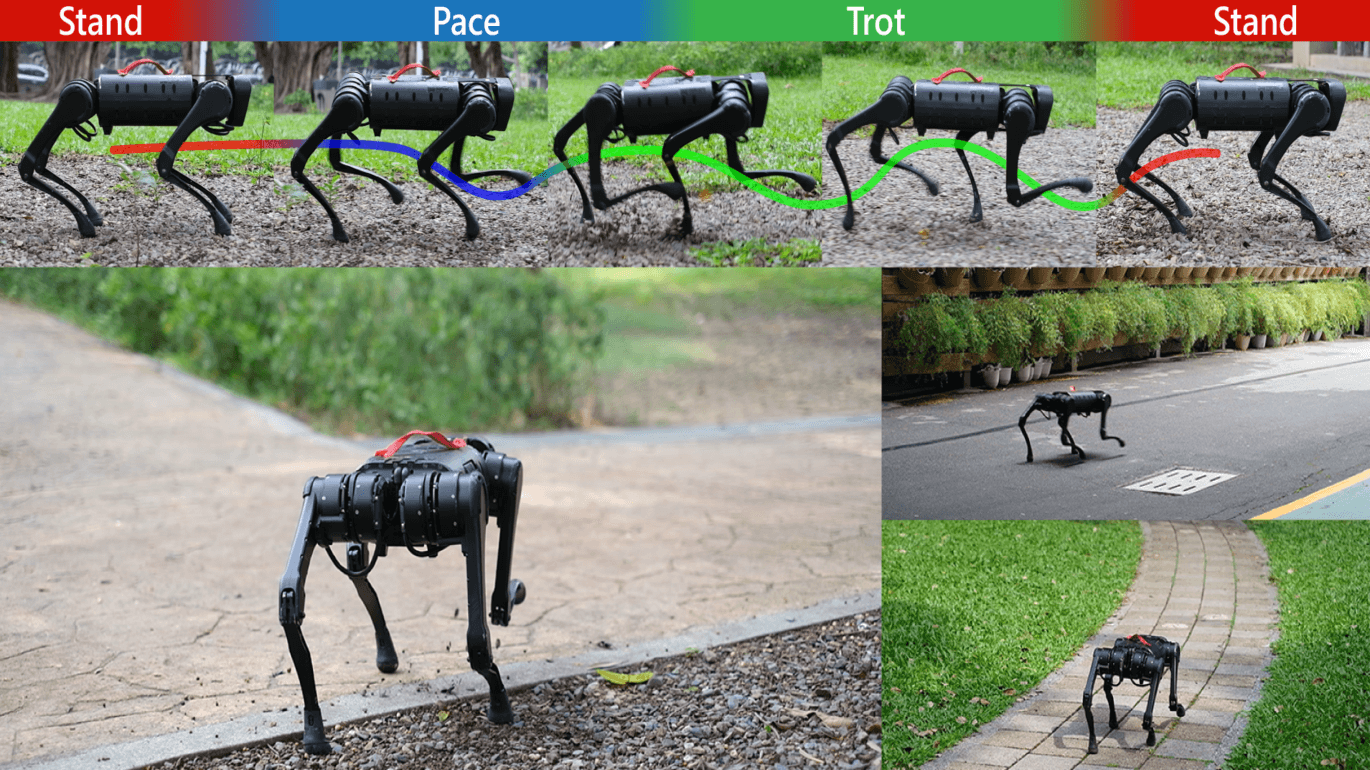
概要
This paper proposes the transition-net, a robust transition strategy that expands the versatility of robot locomotion in the real-world setting. To this end, we start with distributing the complexity of different gaits into dedicated locomotion policies applicable to real-world robots. Next, we expand the versatility of the robot by unifying the policies with robust transitions into a single coherent meta-controller by examining the latent state representations. Our approach enables the robot to iteratively expand its skill repertoire and robustly transition between any policy pair in a library. In our framework, adding new skills does not introduce any process that alters the previously learned skills. Moreover, training of a locomotion policy takes less than an hour with a single consumer GPU. Our approach is effective in the real-world and achieves a 19% higher average success rate for the most challenging transition pairs in our experiments compared to existing approaches.
关键字
- Reinforcement Learning
- Robotics
- Legged Robots
- Control