Dual Deep Learning System to Digitize and Classify 12-lead ECGs from Scanned Images
IEEE 2024 Computing in Cardiology
作者
Chun-Ti Chou, Sergio González
发表日期
2024/9/11
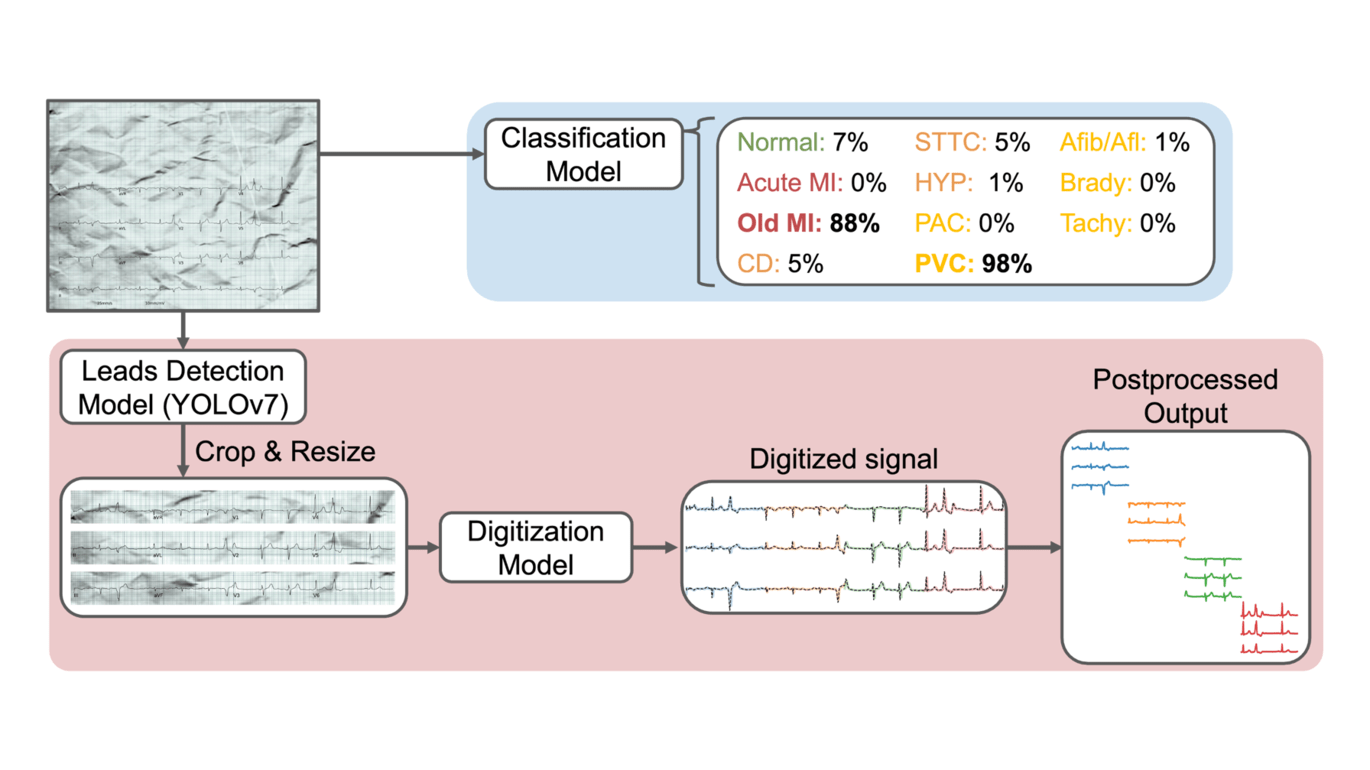
概要
As part of the PhysioNet/Computing in Cardiology Challenge 2024, our team, Inventec AIC, developed a dual deep-learning system to digitize and classify 12-lead electrocardiograms (ECG) from scanned images.
Our approach comprises a computer vision (CV) algorithm and two deep-learning models based on Convolutional Neural Networks (CNN). Our preprocessing algorithm uses contour detection to capture the ECG grid, cropping out non-relevant information and fixing rotations. Our digitization approach leverages a fine-tuned object detection algorithm – YOLOv7 to detect and crop the different ECG sequences. Then, our digitization model based on CNNs with self-attention outputs the digital ECG signals. Besides, we developed an EfficientNet-B0 model with sample weighting to classify ECG images into 11 labels. We trained our models with 336K synthetic images with different formats and distortions from three datasets.
In our internal evaluation, our approaches achieved an SNR of 1.479 and a macro F-measure of 0.728. For the digitization task, our model received an SNR of 0.397 (ranked 21th out of 81 submissions) on the hidden validation set. For the classification task, our model received a macro F-measure of 0.742 (ranked 5th of 90 submissions). Our approach was awarded 2nd place in the classification task of the PhysioNet Challenge.
关键字
- ECG classification
- ECG digitization
- PhysioNet challenge