Domain-Generalized Textured Surface Anomaly Detection
IEEE International Conference on Multimedia and Expo (ICME) 2022
作者
Shang-Fu Chen, Yu-Min Liu, Chia-Ching Lin, Trista Pei-Chun Chen, Yu-Chiang Frank Wang
发表日期
Jul-22
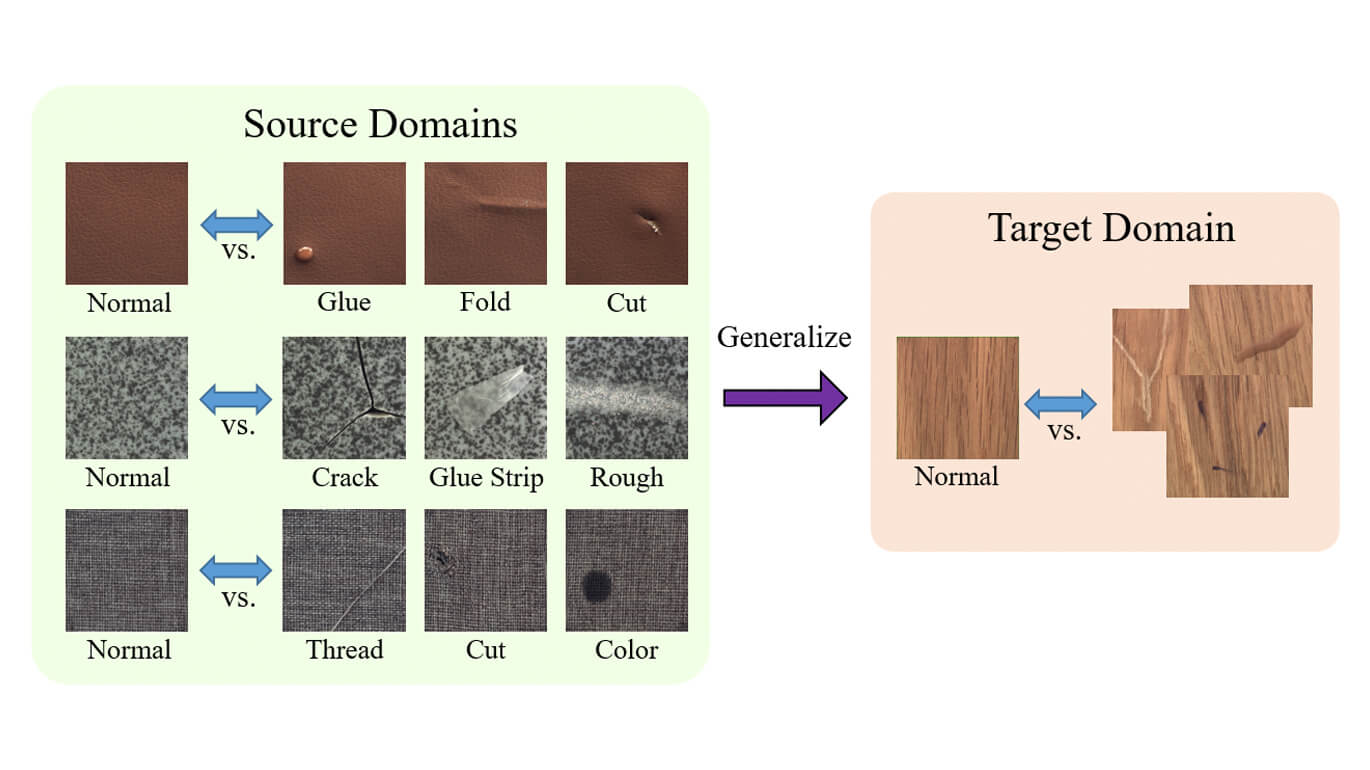
概要
Anomaly detection aims to identify abnormal data that deviates from the normal ones, while typically requiring a sufficient amount of normal data to train the model for performing this task. Despite the success of recent anomaly detection methods, performing anomaly detection in an unseen domain remain a challenging task. In this paper, we address the task of domain-generalized textured surface anomaly detection.
By observing normal and abnormal surface data across multiple source domains, our model is expected to be generalized to an unseen textured surface of interest, in which only a small number of normal data can be observed during testing. Although with only image-level labels observed in the training data, our patch-based meta-learning model exhibits promising generalization ability: not only can it generalize to unseen image domains, but it can also localize abnormal regions in the query image. Our experiments verify that our model performs favorably against state-of-the-art anomaly detection and domain generalization approaches in various settings.
关键字
- Domain Generalization
- Meta-Learning